8 Essential Steps to Successfully Implement Your AI Project
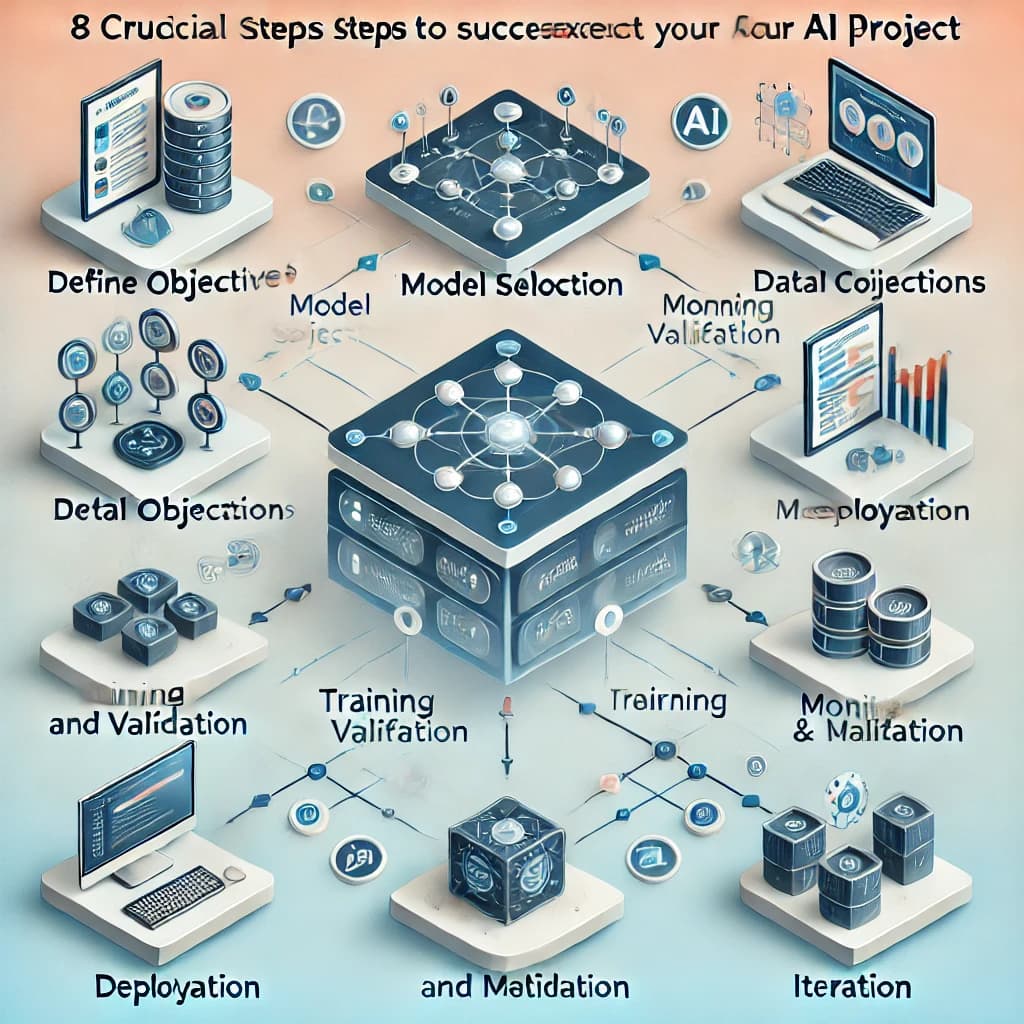
8 Essential Steps to Successfully Implement Your AI Project
Do you have an artificial intelligence (AI) project in mind but don't know where to start? This article presents the 8 essential steps to make your AI project successful. From defining objectives to production deployment, through team building and data processing, discover the best practices for each step.
Key Points
-
Defining clear objectives using the SMART method is essential to guide and evaluate the success of an AI project, highlighting the importance of technology in this process.
-
Building a diverse and competent team is crucial to foster innovation and overcome the challenges of AI projects.
-
Data quality and the preparation and cleaning steps are fundamental to ensure reliable and performant AI models.
Define Your AI Project Objectives
The first step to succeed in your AI project is to define clear and precise objectives. These objectives serve as a compass to guide your efforts and measure the results obtained. Without well-defined objectives, it's easy to lose sight of what you're trying to accomplish and waste precious resources.
Text with incorporated keyword:
Before: New technologies are transforming the economic landscape. Many companies must adapt to remain competitive.
To structure your objectives effectively, use the SMART method, which states that objectives must be Specific, Measurable, Achievable, Realistic, and Time-bound. For example, instead of saying "improve efficiency," you could say "reduce data processing time by 20% in six months." This clarity allows you to choose appropriate technologies and define concrete steps to achieve these objectives.
After the course:
To structure your objectives effectively, use the SMART method, which states that objectives must be:
-
Specific
-
Measurable
-
Achievable
-
Realistic
-
Time-bound
For example, instead of saying "improve efficiency," you could say "reduce data processing time by 20% in six months." This clarity allows you to choose appropriate technologies and define concrete steps to achieve these objectives.
Type: INSTRUCTIONS
Clear objectives also help establish key performance indicators (KPIs) that are used to evaluate the success of your AI project. These KPIs can include metrics such as prediction accuracy, model response time, or user satisfaction. By having well-defined objectives and means to measure their achievement, you can adjust your approach based on the results obtained.
Finally, consider consulting stakeholders to ensure your objectives align with the needs and expectations of all involved parties. This ensures a shared vision and collective commitment to project success.
Define Stakeholders and Target Users
When defining stakeholders and target users, it's essential to understand the needs and expectations of each group. Stakeholders may include company leaders, development teams, end users, and customers. Target users are the people who will directly interact with the system or product.
To define stakeholders and target users, follow these steps:
-
Identify groups impacted by the project: Start by listing the people and groups who will be affected by the AI project. This may include leaders, technical teams, end users, and customers.
-
Understand each group's needs and expectations: Organize meetings or interviews to gather specific expectations and needs from each group. This will help align project objectives with stakeholder expectations.
-
Define roles and responsibilities: Clarify the roles and responsibilities of each stakeholder. For example, leaders may be responsible for strategic decision-making, while technical teams will focus on development and implementation.
-
Establish effective communication channels: Set up clear and regular communication channels to ensure all stakeholders are informed of project progress and changes. Use project management tools to facilitate this communication.
By following these steps, you ensure that all stakeholders and target users are aligned with project objectives, which is crucial for its success.
Establish a Project Plan and Timeline
A well-defined project plan and timeline are essential to ensure the success of an artificial intelligence project. The project plan should include objectives, steps, necessary resources, and deadlines. The timeline must be realistic and take into account time and resource constraints.
To establish a project plan and timeline, follow these steps:
-
Define project objectives and steps: Use the SMART method to define clear and measurable objectives. Identify key project steps, from data collection to production deployment.
-
Identify necessary resources: Evaluate personnel, hardware, and software needs for each project step. Ensure you have the necessary resources to achieve your objectives.
-
Establish a realistic timeline: Create a timeline that accounts for deadlines for each project step. Be realistic about time and resource constraints, and plan for contingencies.
-
Define milestones and key performance indicators (KPIs): Identify important project milestones and KPIs to measure the success of each step. This will allow you to track progress and adjust your plan if necessary.
By following these steps, you can create a project plan and timeline that will help you effectively manage your AI project and achieve your objectives.
Build a Competent Team
Once your objectives are defined, it's time to build a competent team. Here are some points to consider:
-
The success of an AI project largely depends on the diversity of skills and perspectives within the team.
-
A diverse team encourages innovation and creativity.
-
These elements are essential to overcome the complex challenges of AI projects.
Your team should include data science experts, machine learning engineers, domain specialists in each AI application sector, and project managers. Each team member brings unique expertise that is crucial at different project stages. For example, data scientists are essential for data exploration and analysis, while engineers focus on model implementation.
To attract and retain the best talent, it's important to create a stimulating work environment and offer professional development opportunities. Offering continuous training and research opportunities can help maintain team members' interest and engagement.
Finally, ensure each team member clearly understands the project objectives and their role in achieving these objectives. Clear and regular communication allows for quick problem resolution and ensures everyone works harmoniously toward a common goal.
Establish Effective Team Communication
Communication is essential for the success of an artificial intelligence project. It's important to establish effective communication channels within the team to ensure all members are informed and involved in the project.
To establish effective team communication, follow these steps:
-
Set up regular meetings: Organize weekly or bi-weekly meetings to discuss progress, challenges, and next steps of the project. Ensure each team member has the opportunity to express themselves.
-
Use online communication tools: Use instant messaging tools, emails, and online collaboration platforms to facilitate communication between team members. Tools like Slack, Microsoft Teams, or Trello can be very useful.
-
Establish a project management system: Use a project management tool to track tasks, deadlines, and responsibilities. This helps maintain an overview of the project and ensure everyone is on the same page.
-
Encourage collaboration and communication: Create a work environment where collaboration and communication are encouraged. Organize brainstorming sessions and workshops to promote idea exchange and problem solving.
By following these steps, you can improve communication within your team and ensure all members work together effectively to achieve project objectives.
Data Collection and Management
Data collection and management are at the heart of any AI project. Without quality data, it's impossible to develop reliable models. The first step is to identify relevant data sources across various sectors and establish processes to collect this data systematically.
Data quality is crucial for obtaining reliable results. Poor quality data can lead to ineffective models and unreliable results. For example, missing or poorly labeled data can skew model predictions, leading to significant financial losses for the company. It's therefore essential to implement rigorous controls to verify and validate data quality.
The data collection and annotation phase can represent up to 80% of the time and resources needed for an AI project. This step is often underestimated, but it's crucial for project success. Use automation tools to facilitate the collection process and reduce human errors.
For effective data management, adopt secure storage and processing practices. Ensure your data is accessible to team members who need it while protecting sensitive information. Data management must be flexible to adapt to evolving project needs and new data sources.
Data Preparation and Cleaning
Once data is collected, the next step is its preparation and cleaning. This step is crucial to ensure data quality before its use in AI models. Adopt cross-validation techniques for verifying data accuracy and reliability.
Implementing quality control measures is necessary to ensure data annotations are correct and accurate. For example, using specialists for data annotation can help detect errors that would otherwise be difficult for AI algorithms to identify.
Use specialized tools for data preparation and cleaning. These tools can automate error detection and correction, such as missing values and duplicates, and offer suggestions to improve overall dataset quality before analysis.
Data security is also a crucial aspect of this step. Here are some points to consider:
-
Ensure all data cleaning and preparation operations are performed in a secure environment.
-
Protect against data breaches.
-
Avoid loss of sensitive information.
By following these recommendations, you will help ensure your data security.
Finally, data preparation and cleaning must be continuous processes. Data should be regularly checked and updated to reflect changes and new information, ensuring AI models remain relevant and accurate.
Data Exploration and Analysis
Data exploration and analysis are essential steps for discovering meaningful patterns and insights from large datasets. Here are some key points to remember:
-
Data exploration allows transforming raw data into actionable information.
-
This facilitates informed decision-making.
-
Data analysis helps identify trends and relationships within the data.
These steps are crucial for making the most of available data.
Anomaly detection is an important part of data exploration. Identifying outliers can signal issues such as fraud or system failures, allowing for rapid and preventive intervention.
Data exploration techniques continue to evolve, offering opportunities to use real-time data and improve AI models' predictive capabilities. For example, real-time analysis can be used to monitor financial transactions and immediately detect any suspicious activity.
Data scientists play a key role in this step, using advanced data mining techniques to extract valuable insights. In-depth data analysis can reveal hidden trends and correlations, thus helping to refine models and improve their performance.
Modeling and Training
Modeling and training AI models are crucial steps for developing performant and reliable systems. Selecting an appropriate algorithm depends on various factors, including task type, data size, and algorithm complexity, particularly concerning artificial intelligence AI.
Supervised and unsupervised learning are two common approaches. Supervised learning uses labeled data to train models to make predictions, while unsupervised learning discovers hidden structures in unlabeled data.
Deep learning, which uses neural networks with multiple layers, is particularly effective at identifying complex patterns and improving prediction accuracy. This technique is widely used for tasks such as image recognition and natural language processing.
Ensemble methods, which combine multiple machine learning models, can increase prediction robustness. Cross-validation is also essential for evaluating model performance on average across different datasets, thus providing a more reliable measure of their predictive capability.
Model Evaluation and Interpretation
Model evaluation and interpretation are crucial steps to ensure AI models meet defined objectives. Evaluating model performance is essential to determine their effectiveness and relevance to project objectives.
Different evaluation metrics can be used depending on project objectives. For example, a confusion matrix can help analyze model accuracy by comparing actual and predicted results, thus identifying classification errors. It's crucial to choose appropriate metrics to obtain relevant results.
Decision trees and random forests are often used for their interpretable results, facilitating prediction understanding. These methods are robust against outliers and can improve prediction accuracy.
Neural networks, although requiring significant computing resources, excel at learning complex models and are used for tasks such as image recognition and natural language processing. Their ability to handle large and complex data makes them a preferred choice for many AI projects.
Use Visualization Techniques to Present Results
Visualization techniques are essential for presenting AI project results clearly and concisely. Visualizations can help communicate results to stakeholders and target users.
To use visualization techniques to present results, follow these steps:
-
Use data visualization tools: Use tools like Tableau, Power BI, or Google Data Studio to create interactive dashboards and charts. These tools allow presenting data visually and comprehensibly.
-
Create reports and presentations: Prepare reports and presentations to share results with stakeholders. Use graphs, diagrams, and infographics to illustrate key points.
-
Use storytelling techniques: Present results engagingly using storytelling techniques. Tell a story around the data to captivate your audience and make results more memorable.
-
Establish key performance indicators (KPIs): Use KPIs to measure project success and present these indicators visually. This allows stakeholders to quickly understand project performance.
By following these steps, you can use visualization techniques to present your AI project results clearly and engagingly, facilitating understanding and decision-making.
Production Deployment and Maintenance
Production deployment and maintenance of AI models are crucial steps to ensure their continuous performance and long-term utility. Deploying a model to production means it's ready to be used in real environments. This step requires careful planning to ensure the model operates optimally and integrates smoothly with existing systems.
Once the model is in production, it's essential to develop maintenance strategies to adapt the AI to evolving user needs and new data. This may include regular model updates, retraining with new data, and adjustments to improve accuracy and reliability. Proactive maintenance allows anticipating potential problems and resolving them before they affect model performance.
Task automation, including call scheduling, is a key aspect for improving productivity. Using AI in this context allows efficiently managing these processes, thus freeing up time for more strategic tasks.
Edge computing allows processing data at its collection point, thus reducing latency and facilitating real-time decisions. This approach is particularly useful for applications requiring rapid responses, such as security systems or financial services. By processing data locally, not only processing speed but also sensitive data security is improved.
Finally, adopting best practices for AI model maintenance is crucial. This includes continuous performance monitoring, model version management, and detailed documentation of changes made. Rigorous and systematic management helps maintain AI models' reliability and efficiency, thus ensuring their long-term utility.
Ensure Data Security and Privacy
Data security and privacy are essential for artificial intelligence projects. It's important to implement security measures to protect data and ensure its confidentiality.
To ensure data security and privacy, follow these steps:
-
Implement security measures: Use encryption techniques to protect sensitive data. Implement authentication systems to control data access.
-
Establish privacy policies: Define clear privacy policies to ensure data is used ethically and compliant with regulations. Inform users how their data will be used and protected.
-
Use data management tools: Use data management tools to track access and modifications. Tools like Apache Hadoop or AWS Data Lake can help manage and secure data.
-
Establish backup and recovery procedures: Implement regular backup procedures to protect against data loss. Ensure you have recovery plans in case of failure or data breach.
By following these steps, you can ensure data security and privacy in your AI projects, thus protecting sensitive information and complying with current regulations.
Monitor Your Model's Performance in Production
Monitoring your model's performance in production is essential to ensure its effectiveness and efficiency. It's important to establish key performance indicators (KPIs) to measure model success.
To monitor your model's performance in production, follow these steps:
-
Establish key performance indicators (KPIs): Identify relevant KPIs to measure your model's success. This may include metrics like accuracy, recall, F1-score, or response time.
-
Use monitoring tools: Use monitoring tools like Prometheus, Grafana, or ELK Stack to track your model's performance in real-time. These tools allow visualizing metrics and quickly detecting anomalies.
-
Analyze results: Regularly analyze results to identify possible improvements. Use data analysis techniques to understand model performance and identify areas for improvement.
-
Establish maintenance procedures: Establish regular maintenance procedures to ensure model continuity. This may include model updates, retraining with new data, and adjustments to improve accuracy and reliability.
By following these steps, you can ensure monitoring of your model's performance in production, thus ensuring its effectiveness and adaptation to evolving needs and data.
Summary
The success of an artificial intelligence project relies on a series of well-defined steps, each playing a crucial role in project development and implementation. Starting with defining clear and measurable objectives, you lay the foundation for success. Building a competent and diverse team is also essential to bring necessary skills to each project stage.
Data collection and management, followed by preparation and cleaning, ensure that data used to train models is high quality and reliable. Data exploration and analysis allow discovering valuable insights, while model modeling and training are at the heart of developing performant AI systems. Model evaluation and interpretation ensure they meet defined objectives and are ready for production deployment.
Finally, production deployment and maintenance of AI models ensure their continuous performance and adaptability to evolving needs. By following these eight crucial steps, you can maximize chances of success for your AI project and make the most of it.
Remember that artificial intelligence is a constantly evolving field. Stay informed of latest advances and continue learning to improve your skills and optimize your AI projects.
Frequently Asked Questions
Why is it essential to define clear objectives for an AI project?
It's essential to define clear objectives for an AI project as this helps guide efforts and measure results, ensuring the project progresses efficiently. Thus, precise objectives contribute to overall project success.
What roles are necessary in an AI project team?
It's essential to have data scientists, engineers, domain experts, and project managers in an AI project team. These roles ensure a comprehensive and effective approach to artificial intelligence solution development.
How does data quality impact AI projects?
Data quality is crucial for ensuring AI models' reliability; poor quality data can not only compromise model effectiveness but also lead to significant financial losses. It's therefore imperative to ensure data excellence for AI project success.
What are some data cleaning and preparation techniques?
Among data cleaning and preparation techniques, we find cross-validation, quality control measures, and data annotation by specialists. These methods ensure reliable and analysis-ready data.
How can I ensure my AI model remains effective after deployment?
To ensure your AI model's continued effectiveness after deployment, it's essential to perform regular maintenance, update it with new data, and implement edge computing strategies. This will ensure your model remains performant and responds to evolving application environments.
Subscribe to our newsletter
Get the latest updates on AI and automation delivered to your inbox.